
- #Benchmark cpu gpu neural network training android
- #Benchmark cpu gpu neural network training series
The _repr_ of the measurement object returned and are used for Including: label, sub_label, description and env which change

We can change the number of threads with the num_threads argument. Is that PyTorch benchmark module runs in a single thread by default. Representations for printing the results.Īnother important difference, and the reason why the results diverge PyTorch benchmark module also provides formatted string Time per run as opposed to the total runtime like ()ĭoes. Setup: from _main_ import batched_dot_bmmĮven though the APIs are the same for the basic functionality, thereĪre some important differences. Setup: from _main_ import batched_dot_mul_sum TorchMultimodal Tutorial: Finetuning FLAVA.
#Benchmark cpu gpu neural network training android
Image Segmentation DeepLabV3 on Android.Distributed Training with Uneven Inputs Using the Join Context Manager.Training Transformer models using Distributed Data Parallel and Pipeline Parallelism.Training Transformer models using Pipeline Parallelism.Combining Distributed DataParallel with Distributed RPC Framework.Implementing Batch RPC Processing Using Asynchronous Executions.Distributed Pipeline Parallelism Using RPC.Implementing a Parameter Server Using Distributed RPC Framework.Getting Started with Distributed RPC Framework.Customize Process Group Backends Using Cpp Extensions.Advanced Model Training with Fully Sharded Data Parallel (FSDP).Getting Started with Fully Sharded Data Parallel(FSDP).Writing Distributed Applications with PyTorch.Getting Started with Distributed Data Parallel.Single-Machine Model Parallel Best Practices.Distributed Data Parallel in PyTorch - Video Tutorials.Distributed and Parallel Training Tutorials.(Beta) Implementing High-Performance Transformers with Scaled Dot Product Attention (SDPA).Inductor CPU backend debugging and profiling.Getting Started - Accelerate Your Scripts with nvFuser.Grokking PyTorch Intel CPU performance from first principles (Part 2).Grokking PyTorch Intel CPU performance from first principles.(beta) Static Quantization with Eager Mode in PyTorch.(beta) Quantized Transfer Learning for Computer Vision Tutorial.(beta) Dynamic Quantization on an LSTM Word Language Model.Facilitating New Backend Integration by PrivateUse1.Extending dispatcher for a new backend in C++.Registering a Dispatched Operator in C++.Extending TorchScript with Custom C++ Classes.Extending TorchScript with Custom C++ Operators.Fusing Convolution and Batch Norm using Custom Function.Jacobians, Hessians, hvp, vhp, and more: composing function transforms.Forward-mode Automatic Differentiation (Beta).(beta) Channels Last Memory Format in PyTorch.(beta) Building a Simple CPU Performance Profiler with FX.(beta) Building a Convolution/Batch Norm fuser in FX.Real Time Inference on Raspberry Pi 4 (30 fps!).(optional) Exporting a Model from PyTorch to ONNX and Running it using ONNX Runtime.Deploying PyTorch in Python via a REST API with Flask.Reinforcement Learning (PPO) with TorchRL Tutorial.Preprocess custom text dataset using Torchtext.Language Translation with nn.Transformer and torchtext.Text classification with the torchtext library.NLP From Scratch: Translation with a Sequence to Sequence Network and Attention.NLP From Scratch: Generating Names with a Character-Level RNN.NLP From Scratch: Classifying Names with a Character-Level RNN.Fast Transformer Inference with Better Transformer.Language Modeling with nn.Transformer and torchtext.
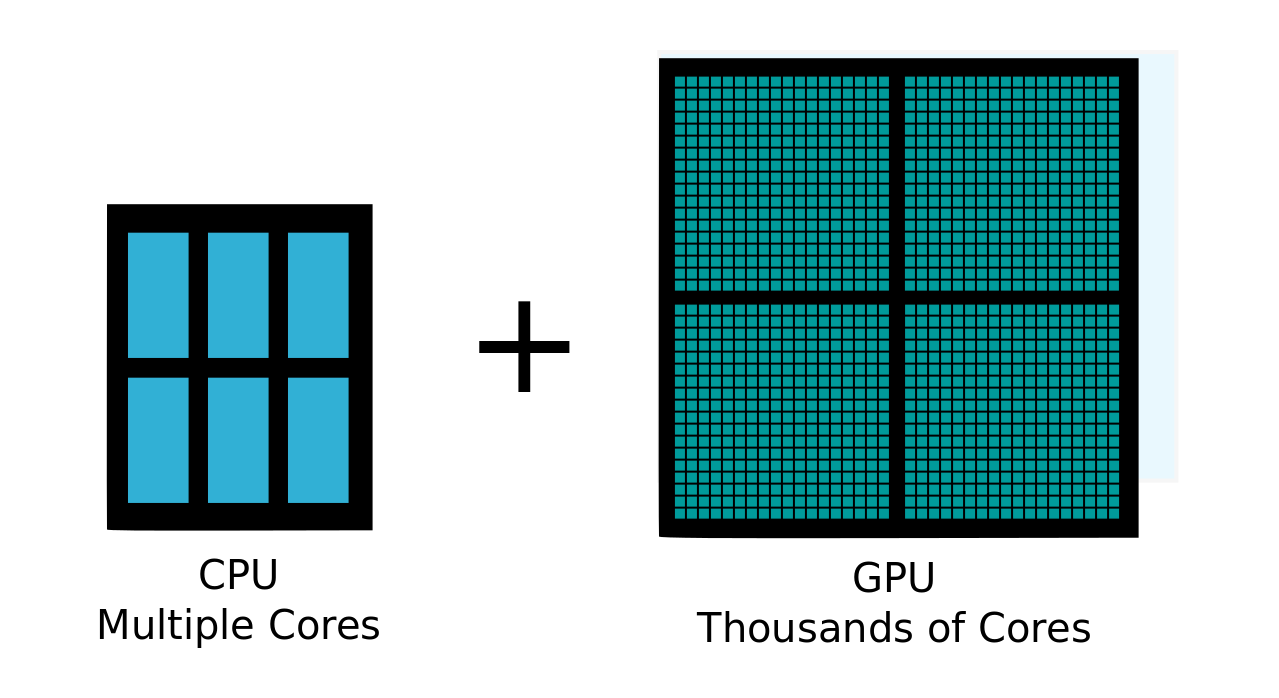
#Benchmark cpu gpu neural network training series
